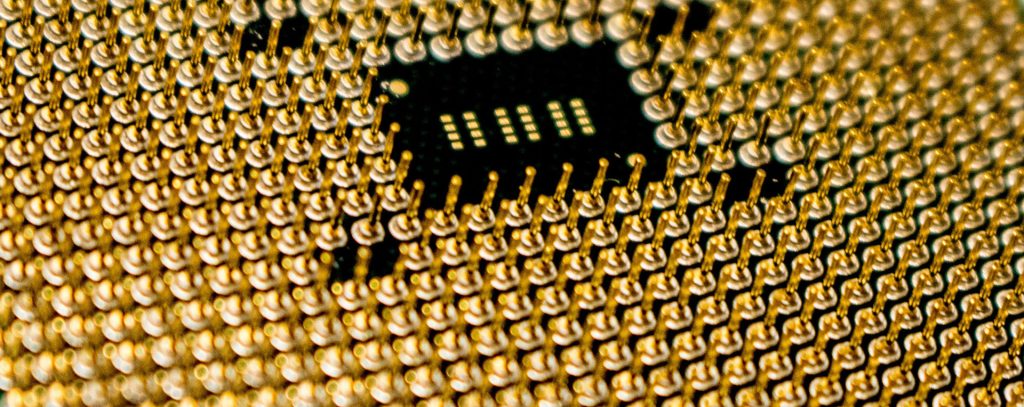
Artificial intelligence (AI) is a rapidly growing field that has gained a lot of attention and hype in recent years. The field has seen numerous breakthroughs and advancements in various applications, from healthcare and finance to gaming and self-driving cars. However, with the excitement and potential benefits of AI also come concerns and risks, such as bias, privacy, and security. In this blog post, we will explore the AI hype and its implications, as well as discuss one of the most prominent AI models, ChatGPT, and its resource requirements, including the relationship between Nvidia ($NVDA) hardware and ChatGPT.
The AI Hype
AI hype refers to the overpromising and exaggerated claims of the capabilities and potential of AI. This hype has been fueled by media coverage, marketing, and investment in the field. Many people believe that AI can solve any problem and revolutionize every industry, leading to the expectation that AI will be the next big thing in technology. However, this hype can be misleading and dangerous, as it can lead to unrealistic expectations, disappointment, and even harm.
One of the main issues with the AI hype is that it overlooks the limitations and challenges of the technology. AI is not a silver bullet that can solve every problem or replace human intelligence and creativity. AI systems are designed and trained to perform specific tasks, and they are only as good as the data they are trained on. Moreover, AI is not immune to bias, errors, and vulnerabilities, which can lead to inaccurate and unfair outcomes, as well as security and privacy risks.
Another issue with the AI hype is that it can create a divide between those who have access to and understand AI and those who don’t. As AI becomes more prevalent and important, it is essential that everyone has a basic understanding of what it is, how it works, and its potential implications.
To avoid falling for the AI hype, it is important to approach AI with a critical and realistic perspective. This means understanding the limitations and challenges of the technology, considering the context and appropriateness of its application, and addressing the ethical and societal implications of AI.
ChatGPT
ChatGPT is one of the most prominent AI models, particularly in the field of natural language processing (NLP). Developed by OpenAI, ChatGPT is based on the GPT-3 architecture and has billions of parameters. It is designed to generate natural language responses to user inputs, such as text messages, emails, and chatbot interactions.
ChatGPT works by using a transformer-based architecture that allows it to learn from a large corpus of text data and generate responses based on that learning. Specifically, ChatGPT is a language model that uses a neural network to predict the next word in a sequence of words. By training on a vast amount of text data, ChatGPT can generate responses that are contextually relevant and coherent.
One of the main advantages of ChatGPT is its ability to generate responses that are similar to human language, making it ideal for use in chatbots, customer service, and other applications that require human-like interactions. Additionally, ChatGPT is designed to be flexible and adaptable, allowing it to learn and improve over time as it is exposed to more data.
However, there are also limitations and challenges to using ChatGPT. One of the main challenges is the computational resources required to train and run the model. ChatGPT has billions of parameters, which requires extensive computing power and memory. Moreover, training and fine-tuning the model can take weeks or even months, depending on the size and complexity of the data.
Resource Requirements for ChatGPT
As mentioned, the computational resources required to train and run ChatGPT are significant. The exact amount of resources depends on the size and complexity of the data, as well as the specific implementation of the model. However, in general, ChatGPT requires a high-performance computing (HPC) system with a large number of GPUs, memory, and storage.
OpenAI, the developer of ChatGPT, has used a range of HPC systems for its research, including those from Nvidia, Google, and Microsoft. In particular, Nvidia GPUs are a popular choice for deep learning applications like ChatGPT, as they provide high-performance computing power for parallel processing and matrix multiplication, which are essential for training neural networks.
For example, OpenAI used the Nvidia DGX-1 system, which consists of eight Nvidia Tesla V100 GPUs with 32GB of memory each, to train the largest version of ChatGPT-3, which has 175 billion parameters. The training process took several months and required petabytes of data and tens of thousands of GPU hours.
While the resource requirements for ChatGPT are significant, they are also becoming more accessible as HPC technology advances and becomes more affordable. Cloud-based services like Amazon Web Services, Microsoft Azure, and Google Cloud Platform offer HPC instances with Nvidia GPUs and preconfigured deep learning frameworks like TensorFlow and PyTorch, which can simplify the process of setting up and running ChatGPT.
Nvidia Hardware and ChatGPT
Nvidia hardware, particularly its GPUs, have played a crucial role in the development and training of AI models like ChatGPT. Nvidia GPUs are designed for parallel processing and are optimized for matrix multiplication, which are essential for training large neural networks.
Moreover, Nvidia has developed a range of software tools and frameworks that can help developers and researchers optimize their AI models for Nvidia GPUs. For example, the Nvidia CUDA parallel computing platform and programming model provides a platform for developing and optimizing GPU-accelerated applications, while the Nvidia cuDNN library provides optimized primitives for deep learning.
Nvidia has also partnered with AI developers and researchers, including OpenAI, to develop hardware specifically designed for AI. For example, Nvidia’s Tesla V100 GPU, which was used to train ChatGPT-3, includes Tensor Cores that accelerate the performance of deep learning operations by up to 12 times compared to previous GPU architectures.
Conclusion
The AI hype has brought much attention and investment to the field of AI, but it has also created unrealistic expectations and concerns. ChatGPT is one of the most prominent AI models, particularly in NLP, and has demonstrated the potential of AI to generate natural language responses that are similar to human language. However, ChatGPT also requires significant computational resources, including Nvidia hardware and software tools, to train and run effectively.
As AI continues to advance and become more prevalent, it is important to approach it with a critical and realistic perspective, understanding its limitations and challenges, and addressing its ethical and societal implications.